An Impending Revolution: Artificial Intelligence Solutions in the Retail Industry
Everybody knows the story of Blockbuster, once the most prominent video rental retailer in the United States. Going to Blockbuster to pick up a movie was an essential attribute of a great Friday night for many of us who grew up in the 2000s. At some point, Blockbuster could have bought Netflix for a mere $50 million.
But the inability of Blockbuster’s management to see the technological future of the industry and their passivity eventually led to the company’s demise. Now, the market cap of Netflix is higher than that of Walt Disney, a company that owns most of the world’s iconic movie and TV brands. What’s the most important lesson that retailers should take out of this story? Innovation, vision, and persistence are the keys to success. Many of the leading retailers have learned this lesson. Many haven’t. Like, Barnes & Noble, once the biggest bookstore chain in North America. In June 2018, their shares hit an all-time low being pushed out by Amazon’s Kindle business. But what stops decision makers at retail companies from driving innovations? Pretty often, it’s the inability to actually understand where those innovations should be applied. Artificial intelligence in retail is a great example. Companies are increasingly adopting solutions provided by AI consultants. Walmart is building out AI capabilities on an unprecedented scale. Kroger is building a model factory, which can rapidly produce hundreds of machine learning models (the foundation of AI) in a short period.
What about other retailers, especially on the global scale? Crickets. Many global, national, and especially regional retailers don’t push AI adoption and severely lag behind.

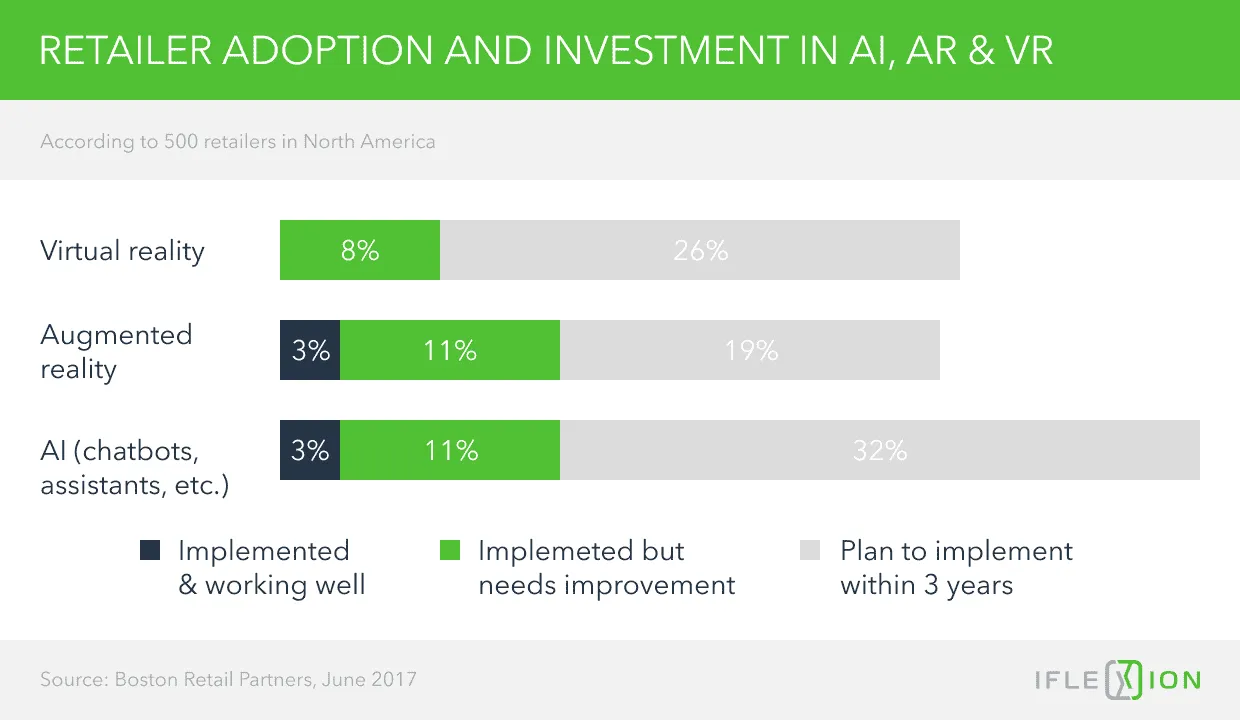
That’s why we wanted to take the opportunity and talk about some of the most profitable applications of artificial intelligence in retail, as well as the functional areas of retail where these opportunities can be discovered and realized right now. These cases are not just about having a fancy team of data scientists and engineers fiddling in their office so that the executives could report on their AI adoption initiatives. These applications have a real business value and ROI.
Endless Opportunities
According to Global Market Insights, the global market of AI applications in retail will grow to exceed $8 billion within the next five years.

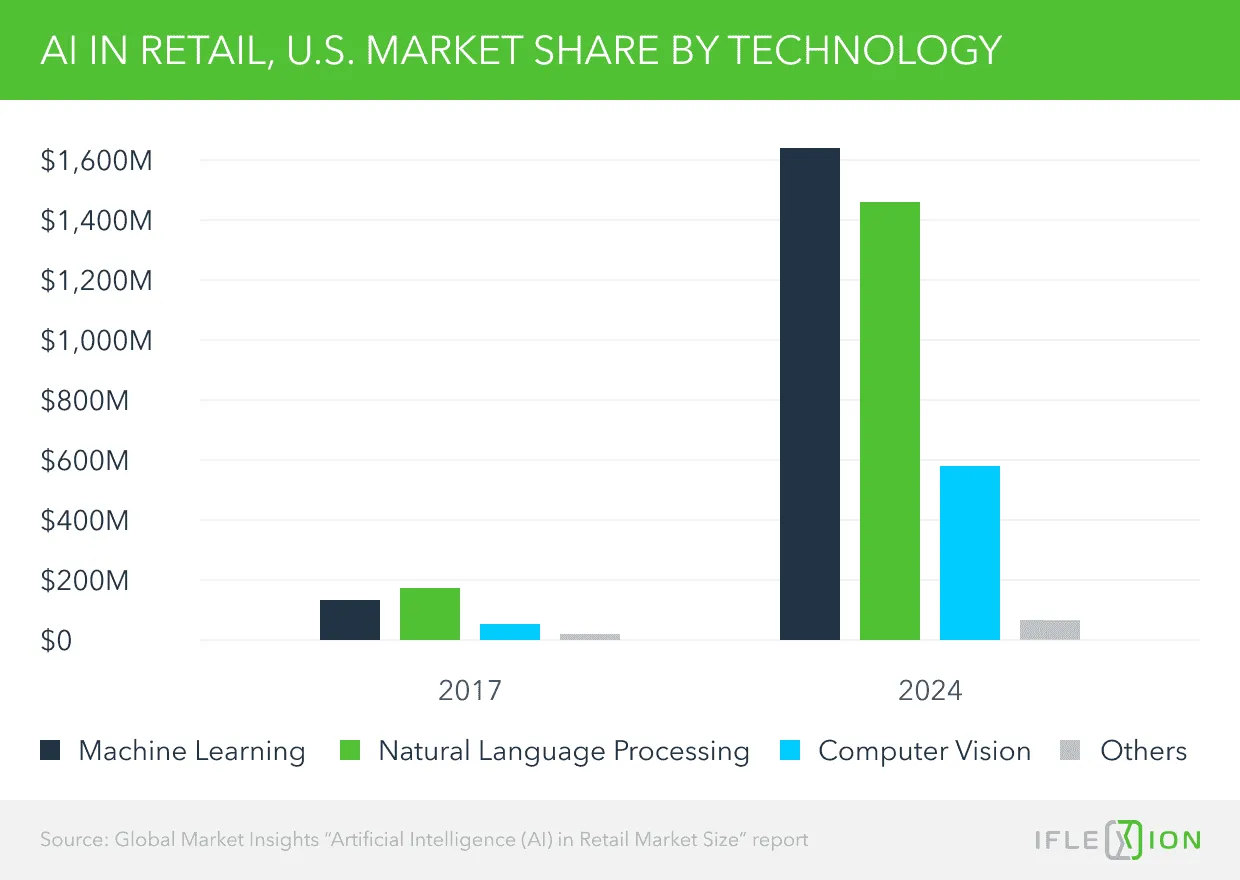
The industry will experience incredible growth because, as we mentioned earlier, retailers are jumping on this bandwagon as soon as they can. Below are some of the major areas that will be affected by these technologies.
Customer & Marketing Analytics
This niche for AI in retail is vital. Retail is a cut-throat business, and, as we exemplified with Blockbuster, competitors won’t give you any chance to recover. That’s why keeping your customers happy is paramount to an efficient retail business.
Sentiment Analysis
Sentiment analysis is about being able to understand how your customers view your brand. The concept is pretty simple: you take the available signals that come in from social media, public forums, and your internal data (like a CRM) and analyze the overall sentiment presented in this data by applying AI to it. In its simplest form, it’s text analysis. On steroids.

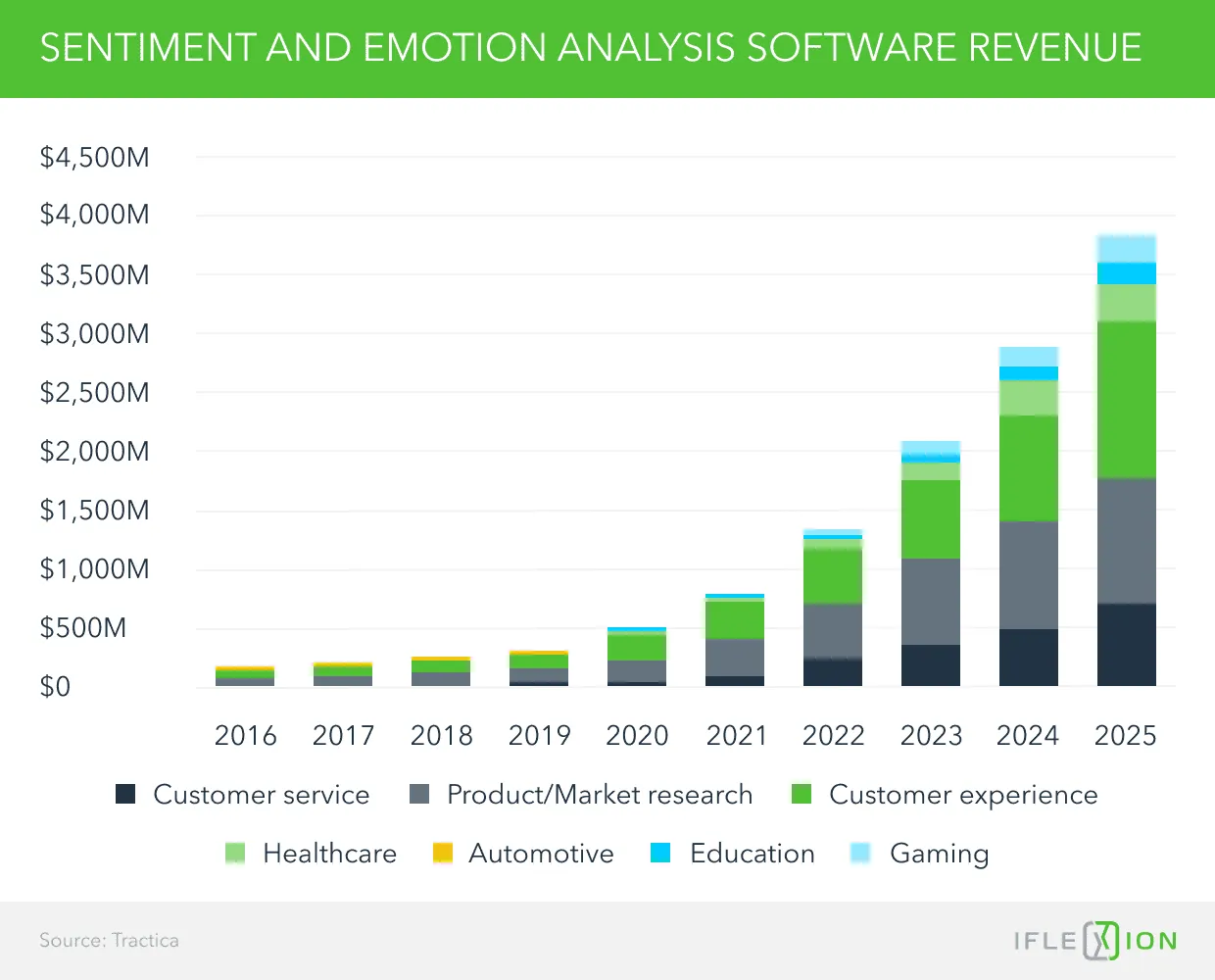
There are a couple of approaches to this task. Luckily, there are open-source tools that can do this. For example, the Stanford Sentiment Analysis framework that uses deep learning to process any signal that you can get your hands on. LongPipe solves the same problem, with its functionality that allows analyzing the polarity of data (how far apart the opinions are), as well as other parameters like subjectivity (how objective the sentiment is). For training, it uses movie review datasets.
Of course, there are commercial tools, like the recently funded Cogito that uses AI to analyze sentiment (in this specific case, for call centers). There are other tools, like Ameyo. It ’s up to you to test them and find out what works and what doesn’t.
Preferences
Being able to predict consumer preferences is very important in driving customer loyalty, as well as inventory and supply chain optimization. When you know what people want, you can adjust your retail inventory to better fit those preferences. You can build whole sets of customer loyalty management activities around this data, like offering coupons to returned customers on specific products that they might be interested in.
This knowledge often transforms the way product recommendations work. In fact, according to McKinsey, around 35% of purchases at Amazon are driven by their recommendation engine. At Netflix, that number is even more impressive. According to the same report, around 75% of the content that people consume on Netflix comes from recommendations. All of these systems are driven by AI—algorithms that analyze previous purchases, search history, wish lists, and other sources to determine the most appropriate products or service offers. You can even take Amazon’s technology to improve recommendations for your retail store. A couple of years ago, they released the source code for their machine learning algorithms that drive product recommendations.
There are, however, commercially available products, like Sentient AWARE, packing similar functionality into an enterprise-ready product.
Targeting
Getting the right message to the right people and at the right time. Seems pretty straightforward? Not really. The devil is in the details. Building a sound market targeting strategy without complex analytics is practically impossible. Sure, you can rely on some basic knowledge about your audience and maybe get lucky from time to time.
But the current complexity of retail interactions and the ways people shop leaves out a lot of the information untouched. This is where AI comes in. AI is perfect for classifying and segmenting potential customers. In fact, classification is one of the classic machine learning problems.
Classifying your customers means putting them in the right “buckets” that you can specifically target. AI can be trained to classify people according to their needs, so every time you’re creating a targeted marketing campaign, it will be aimed at a very narrow ‘bucket’ of users that have the highest propensity to convert through that specific marketing message.

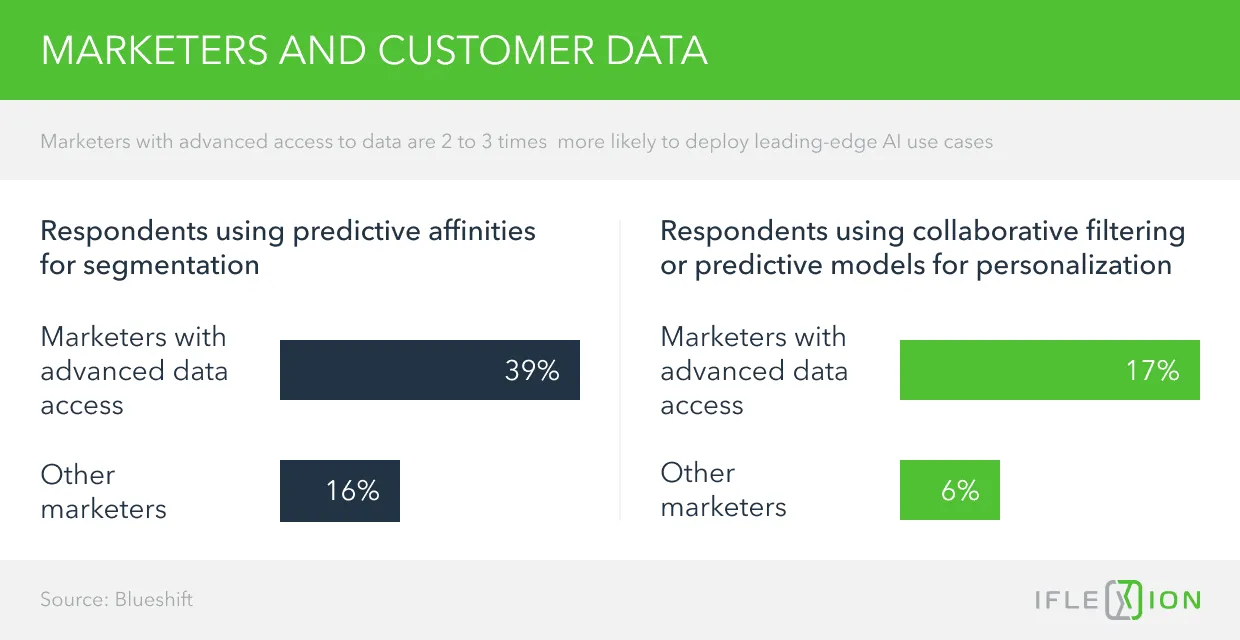
There are commercially available products, like this experiment in the Azure AI Gallery that allows you to segment wholesale customers. There are solutions that you can try building out yourself, like this RFM scoring method that outputs a variety of customer segments (buckets), such as “best customers,” “loyal customers,” etc.
Advertisement Optimization
Programmatic advertising is a huge marketing niche that, while being relatively young, experiences an incredible growth. It may sound complicated, but “programmatic” refers to the basic framework used to buy ad inventory and serve ads through automated auctions or direct purchases from publishers. Google AdWords is the perfect example of that.
According to eMarketer, by 2019 over 80% of the digital advertising expenditures will be focused on programmatic advertising. The same report highlights that over 40% of online ads in 2017 were bought via RTB (real-time bidding).
With the amount of investment in programmatic advertising and RTB, in particular, there are bound to be AI solutions popping up to service them. There are tons of opportunities to optimize bids, ad targeting, and many other parameters (think of all the settings in Google AdWords alone). Machine learning can substantially improve marketing spending once it’s allowed to analyze all this data.
It is a very complex problem with a variety of KPIs, as the accuracy of the system isn’t always the deciding factor in its implementation. RTB auctions during which the fate of the ad space is decided between advertisers, take a fraction of a second. So the AI system has to be both accurate and extremely efficient with its ad bid predictions.
Operations
Being able to operate properly in a fluid market is also crucial for every retailer. That’s why there are a lot of operational use cases that see a successful adoption of AI for complex inventory and supply chain management problems. Cost-effectiveness is everything in the world of incredibly thin margins.
A lot of these problems rely on time series predictions. Time series is just data indexed in time order. So if you have a table with time series data, one of the columns will reflect the time (for example, the time of purchase, e.g., 09:10:22). This is a specific type of AI/ machine learning problem that requires complex data management and processing but also delivers an incredible ROI, as it allows you to estimate a variety of exact operational values, like the exact number of specific items ordered on a specific day in the future.
Inventory & Supply Chain Planning
Being able to predict the optimal inventory is incredibly important. It saves warehouse space; it optimizes resources; it minimizes lost opportunities when a shopper can’t find a specific item that’s not in stock.
There’s a tremendous amount of factors that influence inventory, like the time of day (hello time series), the day of the week, and even weather patterns.

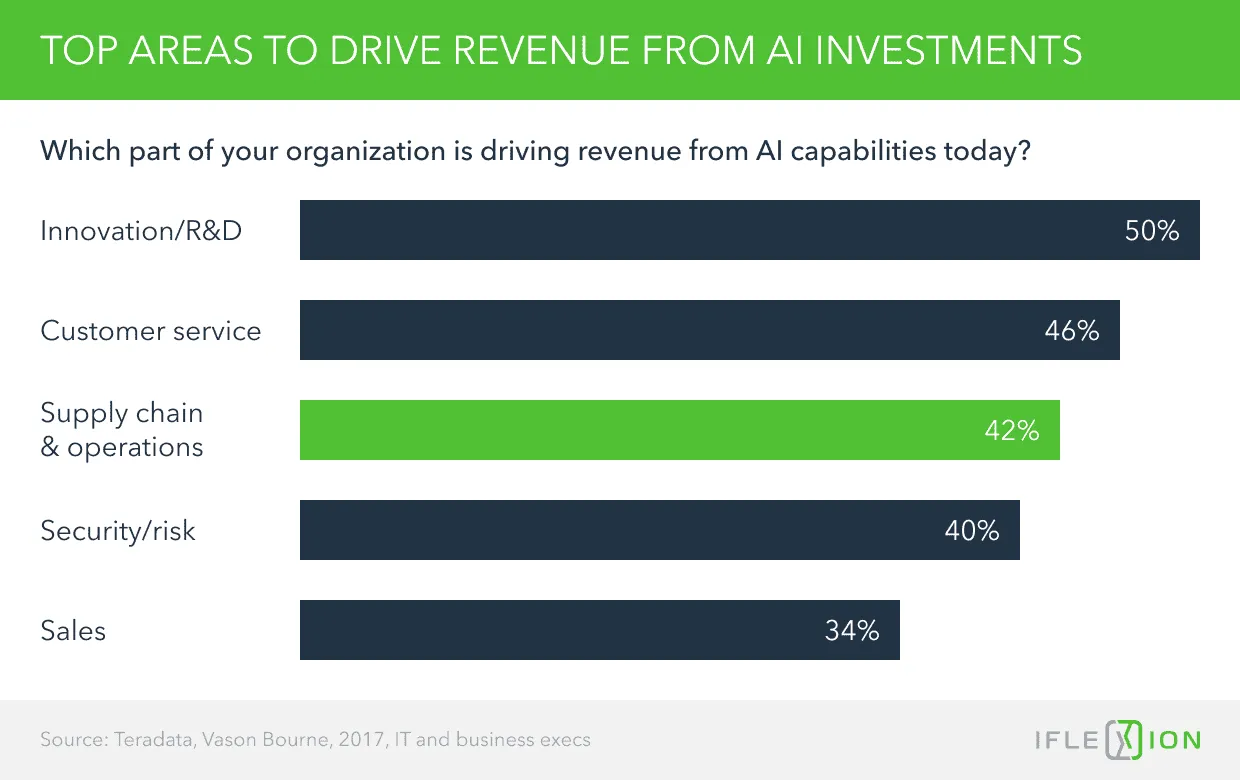
Some AI pioneers are taking it further. Here is Amazon’s anticipatory shipping patent filed back in 2014. The technology allows the retailer to predict what kinds of products will be ordered and from where, so they could ship the order to their distribution center before it’s placed online. When the actual order comes in, they just slap the final address on the package. So yeah, that new pair of skiing pants you wanted to order in a month or two? Amazon already knows about them.
Demand forecasting is one of the cornerstones of these types of supply chain problems. But AI can be used to target even more granular problems within that realm. For example, tools like H2O’s Driverless AI can be used to predict backorders. Machine learning algorithms can be trained to predict product performance for new arrivals, as well as for seasoned products over time. Imagine knowing how much of those green t-shirts will be bought next week? Now multiply that by the thousands of SKUs that you have in your inventory.
There are commercial offerings in these domains, but their enterprise pricing might discourage a lot of people. AI tools like Einstein by Salesforce are used by global corporations to improve their inventory management. There are more flexible solutions, like Frepple, which is an open-source platform for inventory planning with a variety of support plans if you want additional services and functionality. Its basic package is available for free.
Price Optimization
Optimizing the price of your items is a complex task, especially if you have an extensive inventory and serve a rather dynamic market. Dynamic pricing is not a new concept. However, most of the systems that perform these kinds of predictions are either rule-based or rely on outdated algorithms.
AI can easily improve any pricing system through continuous feedback by analyzing historical data and current prices. This is another case that involves time series data and can affect a variety of retail operations—from merchandising (by understanding the seasonality of prices) and product affinities to price sensitivity and promotional cadence. Even if you’re able to improve the accuracy of your pricing through a machine learning algorithm by 1%, it can have a huge impact on your earnings by means of better competitiveness and newly acquired customers attracted by better offers. For example, Bon Prix, a large European retailer, employs AI-based pricing. Their initial implementation of an AI-based pricing system resulted in a double-digit increase in sales, as well as a better profitability per item. These capabilities are enabled by a multitude of products, like PerfectPrice.
It is important to remember that your actual solution will probably depend on your pricing strategy. Are you going for premium, optional or economy market penetration, or any other strategies? It’s highly likely that you might need a custom AI application that can work with your market strategy and pricing goals.
There Is More to AI in Retail
There’s a variety of other applications of AI in retail, which extend well beyond the scope of use cases that we described today. If there’s a process that involves collecting and storing data in your retail business, chances are there’s a ready-made AI solution that can transform the insights that you generate from that data.
A good example is staffing analytics. According to a research conducted by Kenan-Flagler Business School, one of the most reputable business schools in the US, aligning staffing levels with changing in-store traffic patterns can result in a 6.15% savings in lost sales and a 5.74% improvement in profitability for retailers with physical locations. Insights that can help you track and make sense of these patterns can be delivered by AI systems, like those that we mentioned when talking about sales forecasting.
Chatbots are another popular tool for retailers, which promise human-like conversations powered by AI. However, these AI tools are in their infancy. For a conversational AI to be effective, it has to master NLU (natural language understanding) and NLG (natural language generation). These are multi-disciplinary domains that don’t yet deliver highly impactful results. That’s why there are cases when chatbots are already being “fired” by their employers.